Email is still King in Digital Customer Service
.png)
Despite the steep increase in popularity of live chat and instant messaging, specially amongst Millennials, email remains one of the most used communication channels by customers.
The “Email Statistics Report 2019-2023”, published by the Radicati Group, reveals that the worldwide daily email traffic – including all business and personal emails sent and received per day - will exceed 293 billion in 2019[i]. It is predicted to reach 347 billion by year-end 2023, growing at a 4-5% annual rate.
Moreover, a recent survey by Forrester concluded that “email is still the most commonly used digital customer service channel — 54% of customers have used email customer service channels in the last year”.[ii]
To manage the ever-growing number of emails – and other – customer interactions, the usual solution by customer service teams has been to hire additional staff, incurring high costs. However, available automation and AI technology today offer a more economic alternative to contact centre managers with tight budgets to cope with increasing email interaction volumes.
In this blog I will review a few specific cases related to email handling where AI and Machine Learning can help to automate certain tasks and not only keep headcount costs on budget, but also to improve customer service efficiency and response times.
Email Classification and Routing
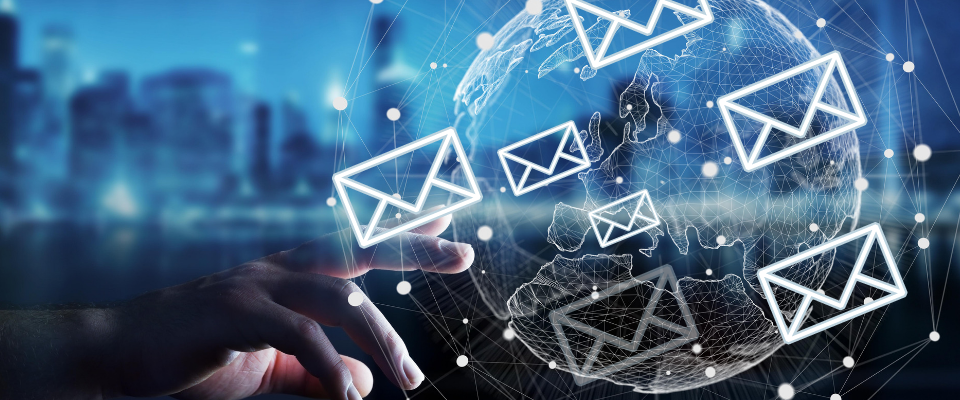
Many of the currently available email management systems use a number of dedicated mailboxes or email queues to identify the service being requested and automatically route the inquiry to the appropriate department within the company. For example, new orders are sent to orders@company.com, while requests for product refunds go to refunds@company.com, and questions about invoices, payments, etc. should be directed to accounting@company.com.
However, in practice many users just write their enquiries to a single generic email address, such as info@company.com or support@company.com, regardless of the nature of their request. In such cases, those emails are first read and analysed by an email pre-processing team, and manually classified and sorted according to the relevant topic or service requested, then routed to the appropriate department (Ordering, Accounting, Refunds, etc) for further action and response.
Despite being a very frequent practice, this represents an unsustainable approach for various reasons:
- Manually classifying emails is a lengthy process resulting in long email response times, which in turn causes customer dissatisfaction.
- It requires keeping a very large staff to perform a manual task which adds little or no value to the end customer.
- Human resources being the highest cost factor in customer service, maintaining and growing such a team quickly becomes economically unfeasible.
Assuming a customer service representative answers on average 50 emails per day, to handle a daily volume of 1000 emails would require a staff of 20 people. That is a huge cost! Available AI technology today can help automate a large part of the process, in particular determining the topic of the email (labelling the request), routing it to the appropriate queue or department, and even sending automated responses without human intervention.
Prioritising Customer Support Tickets
.png)
Let’s now look at another scenario, namely prioritising and assigning customer support tickets received via email.
Usually when a Support Helpdesk Tier 1 person receives a fault report by email, they analyse the information provided by the customer and attempt to confirm the following as a minimum:
- Which system or component is impacted – this determines which Tier 2/Tier 3 technical team or engineer the ticket will be dispatched to for further action.
- The severity of the issue, i.e. the magnitude of the business impact caused by the system fault – this determines the priority for the ticket, how fast the supplier has to react and provide a resolution.
It is not untypical to have a Service Level Agreement (SLA) requiring the supplier to begin Severity 1 (Critical) fault resolution within 30 minutes of having received a fault report. Failing to comply with the SLA might cause the supplier to pay significant penalties, not to mention the damage to the relationship with the customer being affected by the system outage.
In most cases Tier 1 support personnel are not technical experts, therefore they could incorrectly identify the faulty system and assign the ticket to the wrong technical team. To determine the true severity of the problem might also be tricky at times.
Having an AI-powered, self-learning, automated ticket assignment mechanism could help reduce the human error and maintain SLA compliance while ensuring that the problem is handled to the proper technical expert team and fast resolution is provided to the customer issue.
How Can AI and Machine Learning Help
-1.png)
Even if you may not have realised it yourself, email systems already use some sort of artificial intelligence to help you manage your inbox:
- Spam filtering
- Gmail messages being organised into various tabs (Social, Promotions, Updates, etc.)
- Microsoft Outlook classifying your incoming messages by their importance into Focused and Other
All of the above use machine learning and other advanced algorithms in the background to accomplish their task.
Some approaches to automatic text classification use hard-coded rules to determine the topic of an email based on keywords and other relevant elements of the email. However, these methods require deep domain expertise and can easily become very cumbersome, time-consuming and costly to build and maintain.
In contrast, Machine Learning based email classification does not require prior domain knowledge, but it learns to build associations between the content of an email and its relevant topic, by using available email examples, that have been manually classified in the past. Moreover, machine learning methods are usually more accurate than rule-based ones, specially in complex classification scenarios. It is also easier to maintain and it can learn and improve over time as new labelled data becomes available.
Building a Machine Learning Based Email Classification Model
Building a machine learning model for email classification is done in various steps.
Training
In this step, the machine learning algorithm is fed with labelled training samples, so it can learn the associations between the email content and its topic. Assuming that you have archived the past email communication with your customers and those emails were assigned a relevant topic (the label) that would be the perfect training data that is required by the machine learning algorithm.
Testing
In this step we test the trained model performance, i.e. how accurate it can predict previously unseen data. For this purpose, we use a set of sample data – the test set - that was not included in the training set. Typically, the sample data is split 70% for training and 30% for testing.
The topic predicted by the model is compared with the true topic (the label of the test data) and used to calculate the model performance. Multiple iterations of training with different models, parameters and more sample data are usually required until the desired performance goal is reached, i.e. 90% classification accuracy.
Deployment
Once we have a trained classification model that satisfies the performance metrics, it is saved and deployed for use in a production environment. Nowadays, many cloud service providers offer a machine learning platform, including the ability to deploy and use the models from the cloud.
Microsoft Azure Machine Learning services provides an end-to-end platform to develop machine learning models using a large selection of algorithms and tools, including open source libraries. Furthermore, the trained model can be immediately deployed as a Web Service, ready to be used for production purposes.
Such a machine-learning based email classification application can be easily added to most email management systems with minimal or no disruption. The application checks for new emails, runs the classification algorithm in the background and automatically routes the email to the appropriate folder or queue based on the predicted topic or category.
New email data processed and classified by the model can later on be used to re-train the model on a regular basis and improve its accuracy over time.
Figure 1. Building a Machine Learning-based Email Classification Model
Conclusion
Email remains a preferred digital communication channels for many customers and email traffic will continue to increase in the years ahead. Applying AI and Machine Learning technology can help Contact Centres to automate tasks such as email classification and routing, support ticket assignment and prioritisation without the need to hire additional staff and incurring high costs.
Building a machine learning based email classification system only requires a company’s previously processed and labelled email correspondence with their customers. With the use of machines learning cloud services, it has become really easy to train, test and deploy such applications in very short time frames.
To learn more about Geomant AI solutions, please contact us.
[i]https://www.radicati.com/wp/wp-content/uploads/2016/01/Email_Statistics_Report_2016-2020_Executive_Summary.pdf
[ii] https://www.helpscout.com/75-customer-service-facts-quotes-statistics/